Adoption of generative AI requires trust
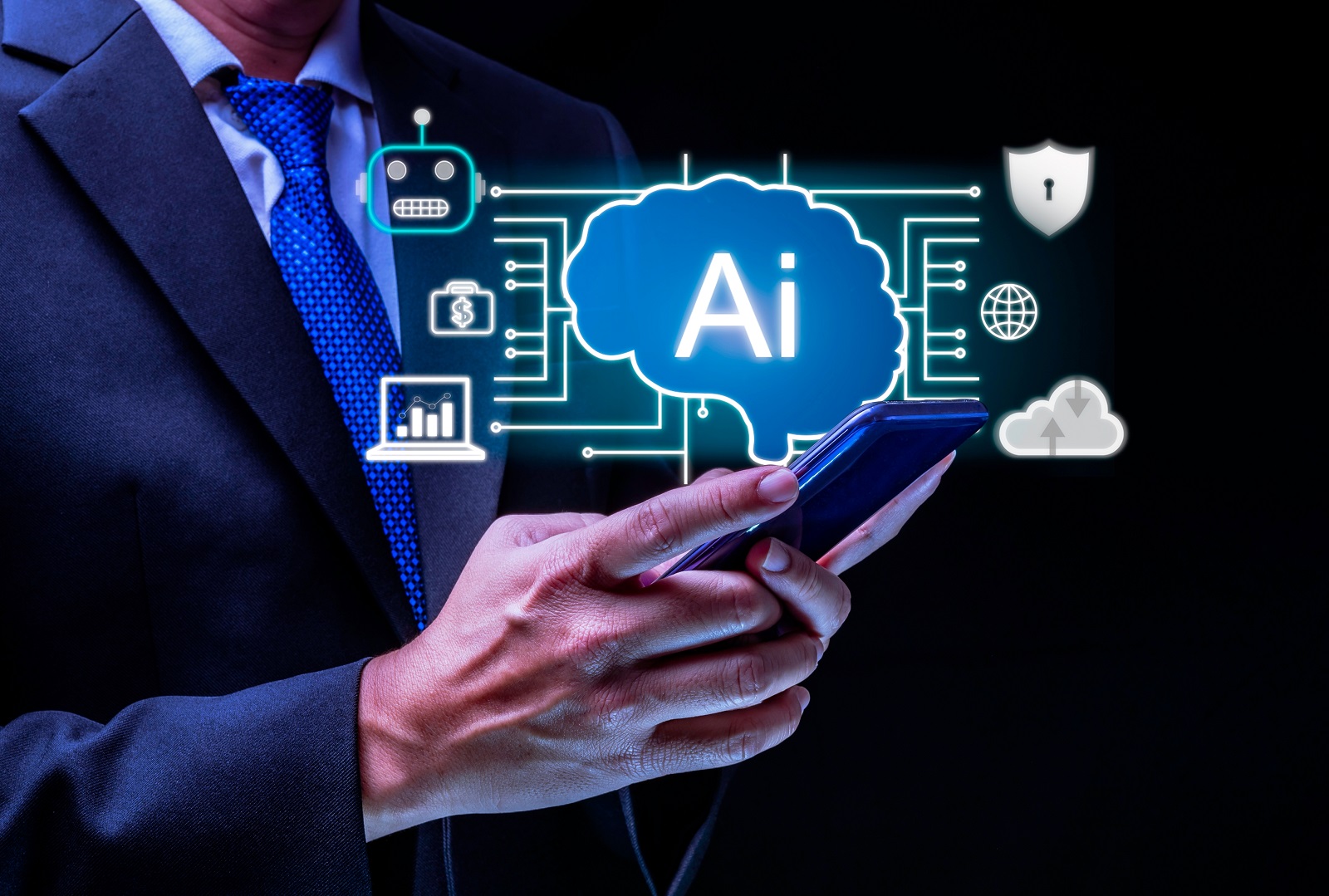
LLM (Large language samples) presents a conversion technology above all. They do not limit themselves to improving existing technology or satisfying a pre-existing need. In fact, generative AI has the potential not only to enrich a company’s products and generate new needs, but above all to fundamentally transform the way individuals work as collaborators and interact as customers.
Reinventing the Concepts of Productivity and Organization
In a classic business process, the approach involves identifying pain points and then proposing a corresponding solution. However, as generative AI brings about a profound change, this traditional approach is coming under greater scrutiny. LLM is not a product in itself, but an engine of change and innovation. Generative AI represents a tool, a capability, that is adaptable to a set of products, services and applications, to improve them, personalize them and make them more efficient. For example, it can be integrated into chatbots to make interactions more natural, into recommendation systems to provide more relevant suggestions, or into data analysis tools to generate specific insights.
In short, it is a technology that requires specific integration and adaptation to specific contexts to exploit its full potential. In a sense, there are no specific predefined use cases, as virtually every aspect of work and business organizations can be affected. Furthermore, when it comes to content-based automation, distinctions between industries become less relevant. However, it is crucial to note that this accuracy does not mean that LLM can accomplish everything, that all clients are the same, or that adjustments are not necessary.
Quite the opposite. Generalist LLMs demonstrate their remarkable ability to digest and generate all types of content. However, there is an opportunity to customize these models to solve sophisticated problems. Each function has specific needs in which it operates, from automated financial reporting to generating product descriptions for e-commerce platforms.
Adapt to business expectations and respond to their needs
Even though OpenAI or Anthropic LLM are extraordinarily powerful, that doesn’t mean they represent the best solution for a particular use case. In reality LLMs need to be adapted and sized to the use case. Thus, RAG (Retrieval Augmented Generation) adaptation techniques make it possible to solve this problem by training the model on the data and referencing it. Then we obtain an LLM that is more efficient than the general model while being more compact and more economical. This customization allows models to tackle complex challenges such as fraud detection, customer relationship management or reading complex contracts. A fine-tuned LLM can be combined with a general LLM to meet different use cases. For example, TheLLMA project embodies an avant-garde initiative in the field of large-scale language models (LLM) specifically dedicated to the French industrial ecosystem.
Ultimately, there is a good chance that similar initiatives will grow in the coming months and years. Successful transformation through AI depends not only on the ability of organizations to identify and use common and fine-tuned models but also on relevant value chains.